Leveraging Natural Language Processing for News-Based Forex Robot Signals
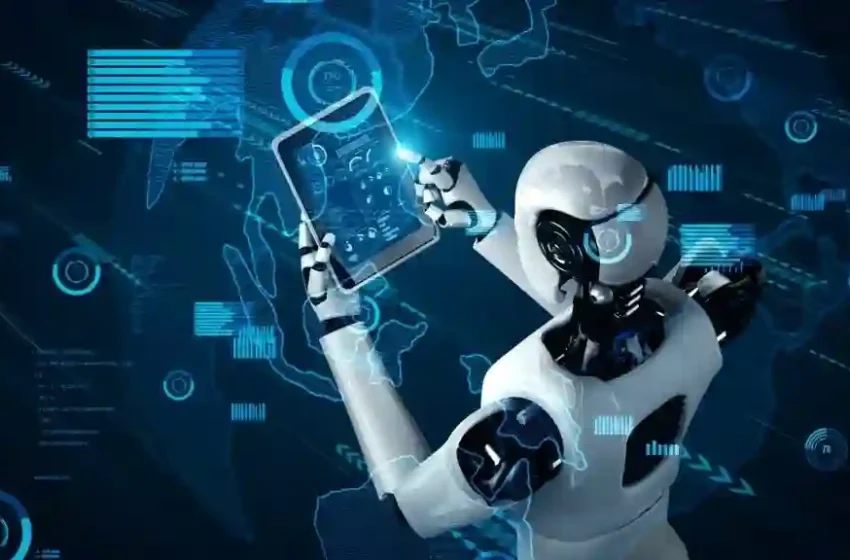
The Forex (foreign exchange) market is highly sensitive to news events and economic indicators, with market sentiment often shifting rapidly in response to breaking news and geopolitical developments. In recent years, advancements in natural language processing (NLP) technology have paved the way for extracting valuable insights from news articles and social media feeds, offering a wealth of information for traders and algorithmic trading systems. This article explores the application of NLP techniques in generating news-based signals for Forex robots, automated trading systems designed to execute trades based on market sentiment derived from textual data. Through a comprehensive examination of NLP methodologies, sentiment analysis techniques, and their implications for Forex trading, this article aims to elucidate the transformative potential of NLP in enhancing trading strategies and decision-making processes in the Forex market.
The Forex market operates 24/5 and is influenced by a myriad of factors, including economic data releases, central bank announcements, geopolitical events, and news headlines. Traders and investors often rely on news sources to stay informed about market developments and make informed trading decisions. However, manually analyzing vast amounts of news articles and social media posts can be time-consuming and prone to human biases. Herein lies the value of leveraging NLP technology to automate the process of extracting relevant information and sentiment from textual data, thereby enabling traders to make data-driven decisions in real-time.
NLP Techniques for Forex Trading:
NLP encompasses a broad range of techniques and methodologies for processing and analyzing natural language text. In the context of Forex trading, NLP can be applied to various tasks, including sentiment analysis, topic modeling, entity recognition, and event extraction. Sentiment analysis, in particular, plays a crucial role in gauging market sentiment and identifying potential trading opportunities based on the sentiment expressed in news articles and social media posts.
One approach to sentiment analysis involves using lexicon-based methods to assign sentiment scores to individual words or phrases and aggregating these scores to determine the overall sentiment of a text. Another approach is machine learning-based sentiment analysis, where algorithms are trained on labeled datasets to classify texts as positive, negative, or neutral based on their sentiment. Deep learning models, such as recurrent neural networks (RNNs) and transformers, have shown promise in capturing nuanced sentiment patterns and extracting actionable insights from textual data.
Generating News-Based Signals for Forex Robots:
News-based signals generated from NLP analysis serve as valuable inputs for Forex robots, enabling them to adapt to changing market sentiment and capitalize on emerging trading opportunities. These signals can take various forms, including sentiment scores, event triggers, and sentiment-weighted indicators, which provide actionable insights into market sentiment and direction.
For instance, a Forex robot may use sentiment analysis to monitor news headlines and social media feeds for mentions of key currency pairs or economic indicators. By analyzing the sentiment of these mentions and aggregating them into a sentiment index, the robot can gauge market sentiment in real-time and adjust its trading strategy accordingly. Additionally, event extraction techniques can identify significant news events and trigger trading signals based on predefined criteria, such as the magnitude of sentiment change or the relevance of the event to currency markets.
Challenges and Considerations:
While NLP technology holds immense potential for enhancing Forex trading strategies, its implementation poses several challenges and considerations. One challenge is the ambiguity and variability of natural language, where the same word or phrase can have different meanings depending on context and tone. Developing robust NLP models that can accurately capture and interpret these nuances requires extensive training data and algorithmic refinement.
Moreover, NLP models may struggle to generalize across different languages, dialects, and cultural contexts, limiting their applicability in global Forex markets. Addressing these challenges requires ongoing research and development efforts to improve the accuracy and robustness of NLP techniques for Forex trading applications.
Future Directions and Concluding Remarks:
As NLP technology continues to advance, its integration into Forex trading strategies is poised to revolutionize the way traders and investors interpret and act on market information. Future research directions may focus on developing hybrid models that combine NLP with other machine learning techniques, such as deep reinforcement learning, to enhance trading performance and adaptability in dynamic market environments.
In conclusion, leveraging NLP for news-based Forex robot signals offers a powerful means of extracting actionable insights from textual data and enhancing trading strategies in the Forex market. By automating the process of sentiment analysis and event extraction, Forex robots can react swiftly to changing market conditions and capitalize on emerging opportunities with precision and efficiency. As NLP technology continues to mature, its integration into Forex trading systems holds the promise of empowering traders with deeper insights and more sophisticated decision-making capabilities in the ever-evolving landscape of global finance.